R3AMA - Robust Reinforcement Learning for floating waste collection
Motivation
In the last decade, Reinforcement Learning (RL) has shown exceptional results on a wide range of tasks, in particular when applied to video games. Most strikingly, these agents have been able to solve both games that require fast, nimble actions, and deep, well-planned decisions. When applied to robotics, these agents have shown similar aptitudes, often outperforming their classical control counterparts. The recent progress in robotic manipulation and legged robot locomotion is a representative example of their capacities on low dimension continuous control tasks. Moreover, the advent of RL, Neural-Networks (NN), and ever-more-efficient edge devices are offering the ability to deploy high-performance visuomotor policies in real time on robots. However, key challenges remain: unlike low dimensions policies, simulators capable of recreating high-fidelity environments are slow, and the appearance of their environments, in particular visual, remains largely different from the real-world. This is notably true for outdoor application, where the complexity of the environment is much higher than indoor. This leads to a gap between what can be simulated and the deployment environments. From these limitations emerge a wide range of problems.
The main developments proposed in the R3AMA ANR project are focused on the development of Reinforcement Learning solutions applicable to real robotic system operating in unstructured environments with real sensors. In particular, RL will be applied to sensorimotor tasks, coordination of tasks on a single agent ad coordination of tasks between agents in a shared environment. The core tools supported these contributions will be model-based reinforcement learning and high-performance, high-fidelity simulation environment. The project demonstrations will address two use-cases: the automation of robotic boats used for waste collection and pollution control and the automation of space debris capture and de-orbiting with robotic satellites.
Autonomous Surface Vehicle for Waste Collection on the Water Surface
A fully autonomous system for collecting floating waste requires the integration of robust perception and control components. The following sections present our approach and initial results.
Perception
Detecting floating waste is challenging due to dynamic lighting conditions and reflections on the water surface. To address this, we utilize a polarimetric imaging system that enhances the contrast of floating objects.
This work is available through the following article:
- Batista, L. F. W., Khazem, S., Adibi, M., Hutchinson, S., & Pradalier, C. (2024). PoTATO: A Dataset for Analyzing Polarimetric Traces of Afloat Trash Objects. Proceedings of the IEEE/CVF European Conference on Computer Vision TRICKY Workshop.
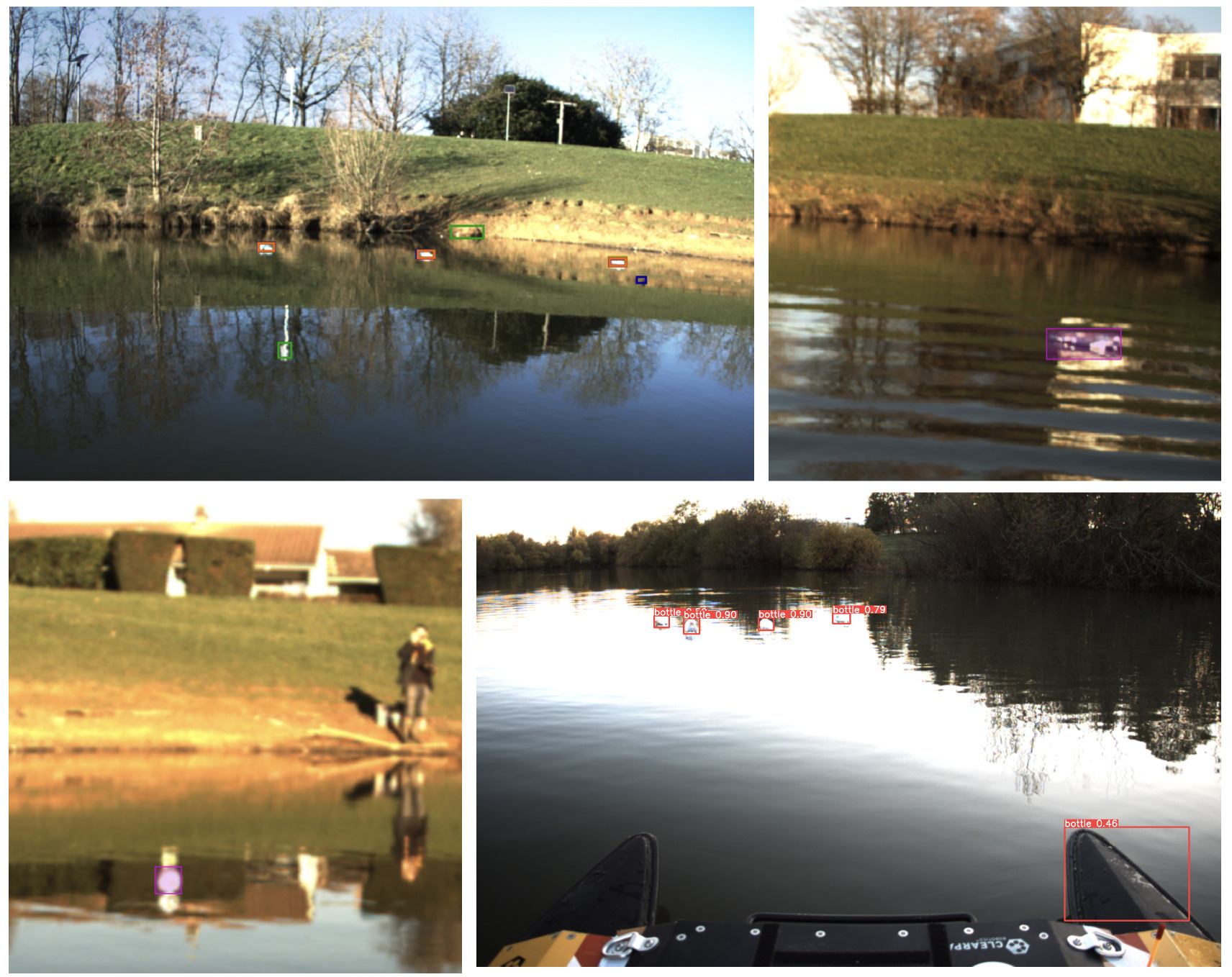
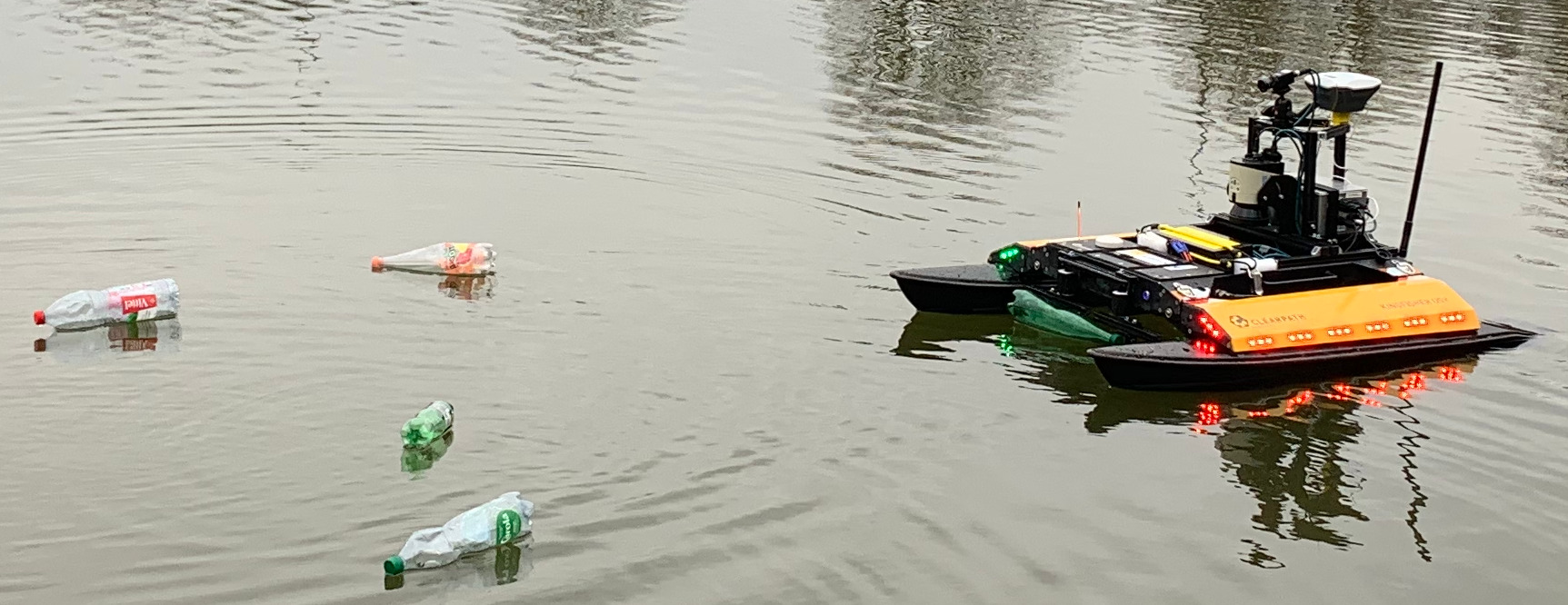
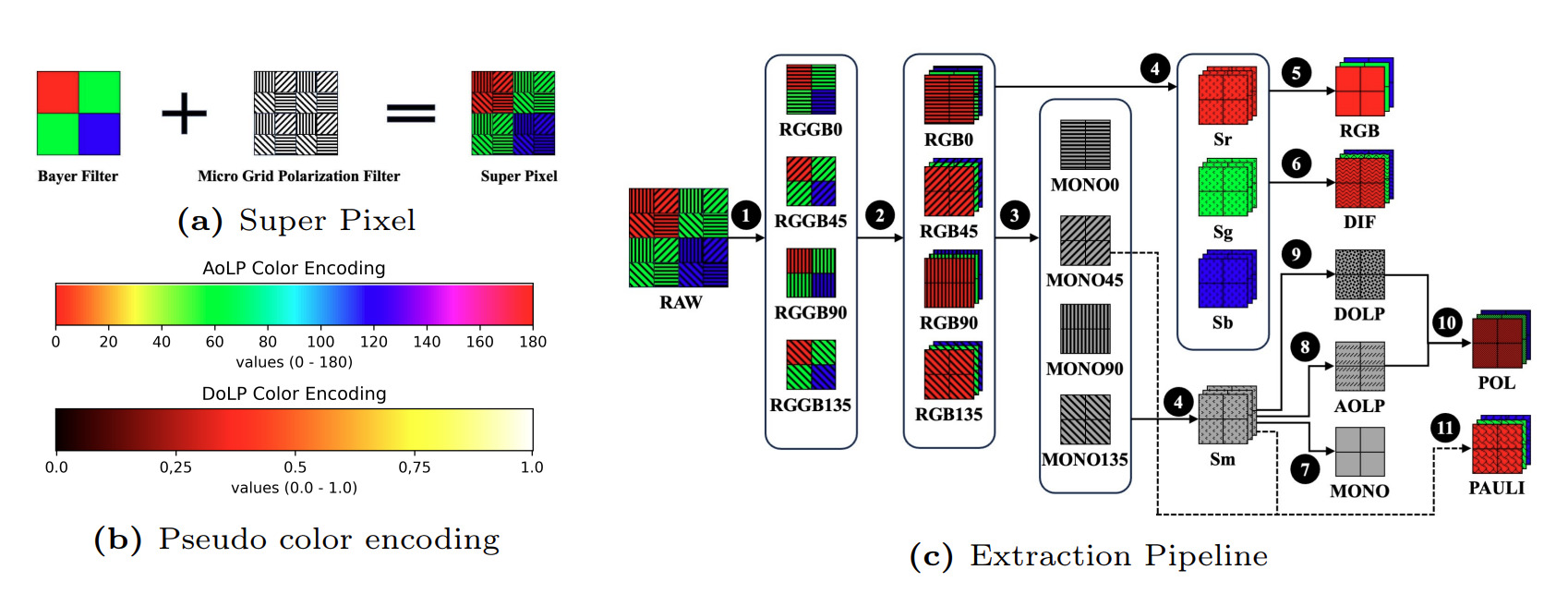
Control
Autonomous Surface Vehicles (ASVs) are affected by environmental disturbances such as wind, water currents, and variable payloads as waste is collected. To ensure robust navigation under these conditions, we employ a deep reinforcement learning (DRL) framework.
This work is available through the following article:
- Batista, L. F. W., Ro, J., Richard, A., Schroepfer, P., Hutchinson, S., & Pradalier, C. (2024). A Deep Reinforcement Learning Framework and Methodology for Reducing the Sim-to-Real Gap in ASV Navigation. 2024 IEEE/RSJ International Conference on Intelligent Robots and Systems (IROS), 1258–1264. https://doi.org/10.1109/IROS58592.2024.10802067
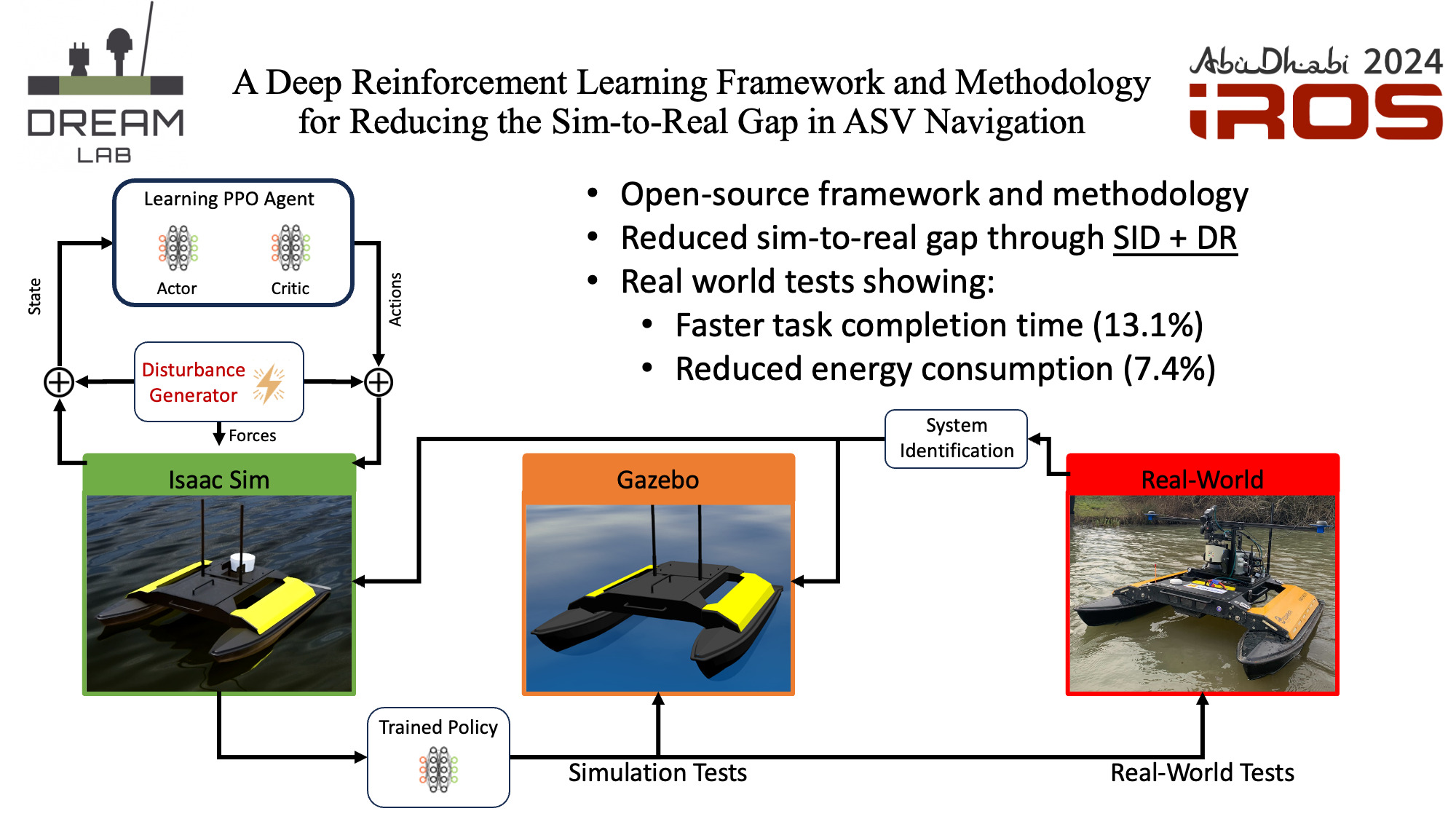
Project Partners:
- CNRS IRL2958 GT-CNRS [FR]
- Joint research lab involving CNRS and the Georgia Institute of Technology
- Expertise: Field Robotics, AI, Robotics for Natural Environments
- Cédric Pradalier, Stéphanie Aravecchia
- IADYS [FR]
- SME commercializing Unmanned Surface Vessels (USV) for waterway cleaning
- Expertise: USVs, System Integration, Computer Vision
- Nicolas Carlesi, Ronald Loschmann
- University of Luxembourg [LU]
- Space Robotic laboratory
- Expertise: Space Robotics, AI, RL, Zero G environment
- Miguel Olivares-Mendez, Antoine Richard
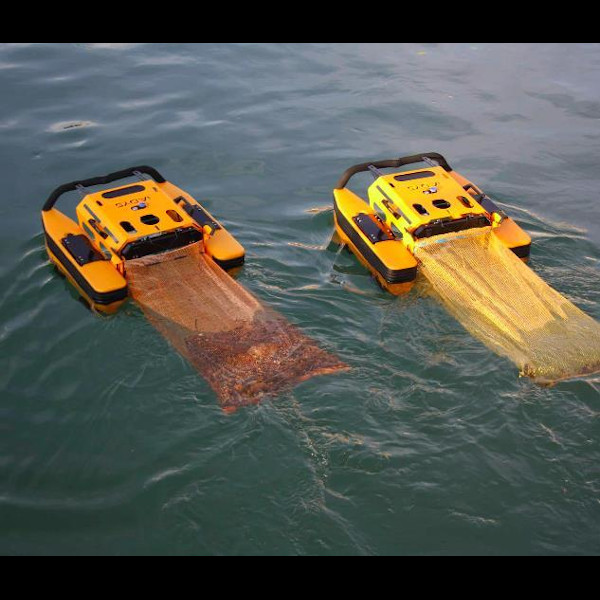
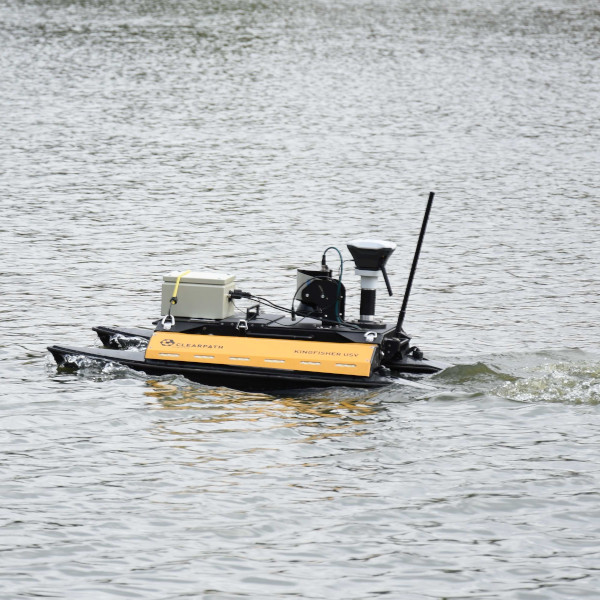
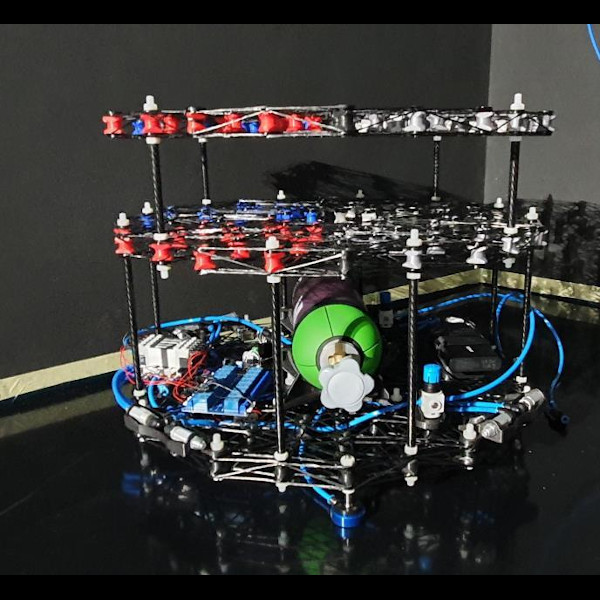